AI and OSINT: New Breakthroughs Meet Next Gen Solutions
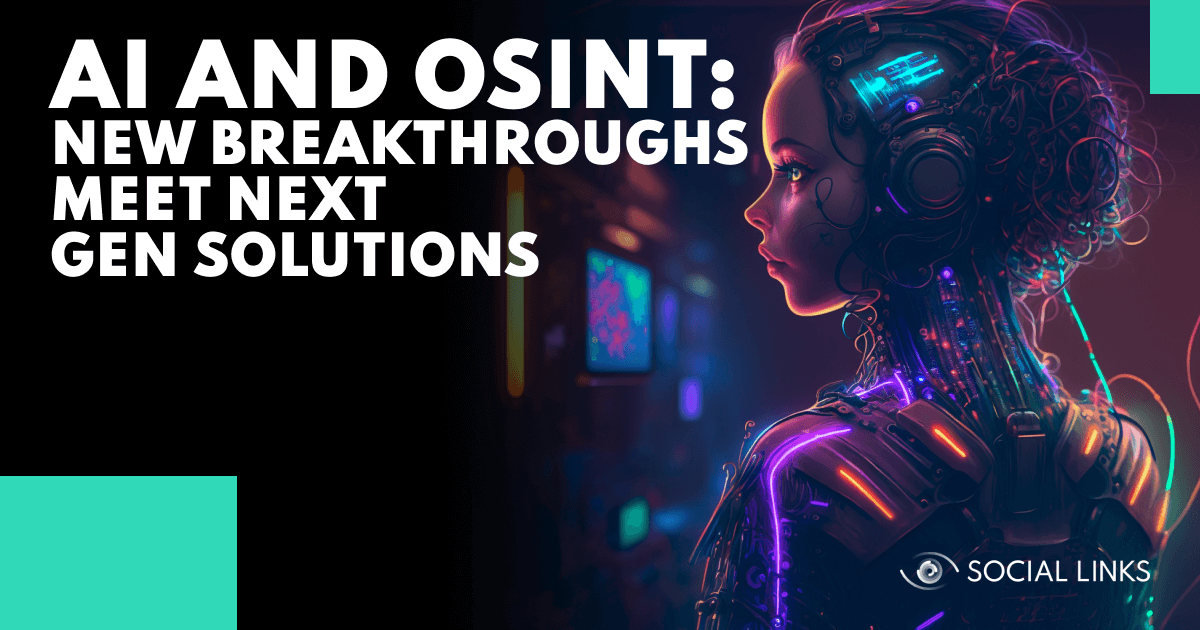
Whether it’s virtual assistants, personalized shopping, chatbots, content recommendations, or any number of other preemptive operations, you can scarcely use your smartphone without invoking some kind of AI algorithm. While the omnipresence of machine learning is a testament to its power and utility, the term has not been without its gimmicky side. For instance, Oral-B’s $220 AI-powered toothbrush raised a few eyebrows.
But in the last year, there have been developments afoot that have brought AI tech firmly back to center stage. Systems of groundbreaking capabilities such as ChatGPT, Midjourney, and Dall-E 2 have made a splash, causing many to suggest that we could be on the threshold of a technological paradigm shift. If so, a serious question for Social Links is: where does OSINT fit into all of this?
So, in this article, we’re discussing what the role of AI technologies has been in open-source intelligence over the years, and how some of the very latest developments in machine learning are already becoming part of the most advanced OSINT tools.
The recent emergence of these remarkable, generative AI systems has fueled projections about the global market, which is shaping up in a significant manner. With a registered CAGR of 38.1%, market size is expected to balloon from 2022’s $119B to a staggering $1.59T by 2030. However, there’s more than mere hype driving these projections—growth seems to be happening in tandem with that of another market: cyber security.
With cyberattacks estimated to be costing organizations $10.5T annually by 2025—triple the figures of 2015—cyber vulnerability represents a spiraling global concern. At the same time, cybersecurity solutions on the market are falling short of customer needs in terms of their levels of automation and pricing. According to McKinsey, this shortfall equates to a tantalizing $2T gap in the market for vendors of cybersecurity solutions.
AI is the lifeblood of fast and effective automation. Since these frontier technologies are starting to display abilities that border on sci-fi, it’s reasonable to assume that the projected growth of the global AI and cyber security markets is more than just coincidence. And OSINT tool developers will be right in the thick of it—at this intersection of demand and possibility, a new chapter in open-source intelligence is set to begin.
But let’s rewind a bit. The relationship between AI and open-source intelligence is not a new phenomenon. In fact, the two have been inextricably linked for decades.
In the advent of the World Wide Web, open data took on an entirely new meaning. As an unprecedented proliferation of digital data poured forth, OSINT—once the analog province of newspaper publications and TV broadcasts—was reborn. For analysts, the explosion of open data brought with it a world of opportunity. Equally, it posed immense challenges.
So, we had data. And lots of it. And we knew this data could be channeled to solve any number of questions and complete all kinds of tasks. But to achieve this, needles needed to be found in the haystacks, or patterns coaxed out of oceans of data. As can be easily imagined, the tools and techniques applied pre-automation were totally overwhelmed, and all the while, the amount of data just kept exponentially growing. In the last decade, online data has exploded from approximately 7 zettabytes to an enormous 97.
Enter AI. Realizing that algorithms could do a lot of the legwork, developers began creating AI models whose parameters could be altered in accordance with the task at hand. Leaving the system to extract or filter the data in the way they needed, analysts could get on with the decision-making that facilitated the overall process, and reach the objectives faster.
As the volumes of data have expanded, machine learning models have developed in symbiosis, to the extent that entire OSINT disciplines would now be inconceivable without AI assistance. In fact, thanks to AI, the scale of the open data universe is no longer an insurmountable challenge, but an advantage—one that is revolutionizing information analysis in various ways.
When it comes to the intelligence cycle, one size doesn’t necessarily fit all, and models can vary depending on the objectives and workflows of a given organization. But broadly speaking, there are six overarching stages, which are generally accepted as the main beats of the process. These are: planning & direction, collecting, processing & exploitation, analysis & production, dissemination & integration, and evaluation & feedback.
AI has something immensely valuable to offer each and every one of these stages. So, let’s take a look at the factors that can work against an effective intelligence process, and how modern AI-driven solutions can tackle these obstacles.
This is the beginning and end of the cycle—the stage that closes the loop. It’s there at the start for setting objectives and requirements for the subsequent cycle stages, and it’s there at the end since completed intelligence necessitates action and inevitably informs the next round. Involving management of the full intelligence enterprise, this process is largely shaped by the end-user.
Obstacles. Planning and direction are typically very onerous and time-consuming procedures, especially in large institutions such as public sector organizations. Departments often have their own procedures and intelligence policies, while a single workflow is rather rare. Moreover, analysts frequently have to restart the intelligence process from the initial stage due to poor elaboration during the planning stages.
AI Solutions. Short, automated, multi-stage intelligence cycles. By splitting big intelligence cycles into smaller ones, project coordinators can quickly tune the workflow before the final dissemination of the intelligence insights. However, in order not to overload the whole process, the lower-tier cycles need to be maximally automated.
Tools. There are currently no fully automated short intelligence cycle management tools on the market, however, such products are expected to arrive soon as add-ons to more advanced investigation systems.
Collection consists in gathering and recording raw data—the basic ingredients to be refined into actionable intelligence. This data can come from almost anywhere including open sources such as social media, online forums, public records, articles, and essays, as well as closed sources like CCTV footage, interviews, and so forth. Private sector units tend to be broad and versatile in their collection approaches; public sector bureaus are more focused and specialized.
Obstacles. The huge amount of user-generated information on the internet and its various formats (text, audio, video, and image), have made it practically impossible to effectively collect all necessary information manually. Furthermore, international investigations require the use of different languages that cannot be efficiently translated with standard online translation tools.
AI Solutions. Some OSINT systems provide ML-based information-gathering features. With different parameter setting options, these tools can automatically search for all manner of information that can be correlated with the initial input. Such systems may also include AI-driven translation plugins allowing users to reconstruct nuanced text semantics across multiple languages.
Tools. Deepmind is a prominent example of an ML-driven language model. Automated information collection is offered by some OSINT systems, including SL Professional.
The processing stage is all about getting the unrefined information into a suitable condition for the analysts to start doing their thing. This involves indexing, grouping, and structuring the raw data, and may also include the digitization of all analog information relevant to a case. Processing doesn’t happen in isolation as a closed step in the cycle but fluidly overlaps with much of the collection and analysis work.
Obstacles. The processing of data is no less challenging than its collection—and for similar reasons. While many intelligence systems already excel at textual analysis, other data formats such as audio, image, and video, still present difficulties. And due to the spread of deepfake technologies, it is becoming increasingly tough to separate the relevant information from the noise.
AI Solutions. Although still in their infancy, there are some promising AI-enabled systems for processing non-textual information. These solutions include functions such as voice recognition, image reconstruction, and deepfake detection.
Tools. Neural networks from the GPT family can effectively analyze large amounts of text information but are not so adept at recognizing deepfakes, fake news, or other AI-generated content. Fortunately, there are tools that can do this well—Writer, Deepware, and Sensity, to give three examples. However, AI-generated texts can be seriously challenging to flag up. If an AI-generated text receives human editing, the likelihood of identification plummets to 25%.
This is the stage where the information graduates from the status of mere data to that of fully-fledged actionable intelligence. Broadly put, it is a process of data integration and assessment to produce coherent intelligence material. The analysts doing this work are trained specialists who evaluate the data in terms of how trustworthy, valid, up-to-date, and relevant it is. The data is combined into coherent amalgams, contextualized, and sculpted into usable intelligence complete with event breakdowns and analyses, as well as the implications these may have for the end-user.
Obstacles. Even after being processed, data can be disjointed, jumbled, and conflicting. Add to this the fact that it could be pouring in by the terabyte and you can get a picture of just how challenging the work of the analyst is. As online data generation continues to blow up, it is becoming even more difficult for analysts to find links among the masses of multi-source information and derive valuable insights. In fact, analysts are often forced to neglect considerable amounts of information, due to the sheer impossibility of fusing it all into a single, logical report.
AI Solutions. AI-driven graph analysis tools are already on the market and have become staple solutions in modern investigations.
Tools. There are a number of powerful link analysis systems out there, including SL Professional, with its advanced graph analysis algorithms. For textual analysis tasks, ChatGPT-based models are proving effective, while for vocal analysis, there are systems that can extrapolate the vocal data from a three-second sample and then match it with different audio to identify speakers. Meanwhile, Nvidia’s audio2face tool can even generate photorealistic portraits from vocal recordings and then find the speaker on social media via facial recognition. A remarkable feature, and one of great interest for digital forensic analysts.
Once the intelligence has been derived, it of course needs to be communicated to the end-users—those who commissioned the work in the first place. This part of the process typically triggers some form of feedback, which will often be the seed of a new cycle. Clarity of communication is essential at this stage, and different clients will require different formats including written reports, briefings, and PowerPoint presentations.
Obstacles. There’s an overriding difficulty in that, often the same qualities that make a first-rate analyst can also make a third-rate communicator. Whatsmore, analysts generally find this stage of the cycle painful and dreary due to its routine nature, which impacts the quality of intelligence presentation. It’s not uncommon that analysts are scolded by their superiors for turning over poor-quality reports that suffer from improper language style, jargon, inconsistency, and lack of logic.
AI Solutions. AI systems that can help analysts structure, summarize and correct rough drafts of intelligence reports are a clear way to address this issue. In helping analysts sidestep a lot of the routing generative reporting while also providing reliable auto-checking, reports can be produced to a higher quality in less time.
Tools. ChatGPT is an obvious solution for this task. For example, in a recent update of SL Professional, the chatbot is harnessed to provide a summarization feature.
Having delivered the intelligence, the bureau doesn’t just wash its hands of the whole thing; dialogue with the client needs to continue. Analysts ultimately need to plug any gaps that may have slipped the net, or more generally get a handle on how well the intelligence met the client’s needs. By making final additions or tweaks, analysts can further satisfy a customer, while reflecting on shortcomings can improve internal processes for future work.
Obstacles. Feedback is often neglected and in most cases turns out to merely be a set of cursory commentaries on the report. Ideally, feedback should be as insightful as the report itself so that the intelligence cycle can self-improve with each new iteration.
AI Solutions. As with the reporting stage, automated textual structuring can be of great assistance to this work.
Tools. Again, ChatGPT is a pertinent solution as it can help intelligence specialists compose text within a well-structured framework preset, ensuring that no important pieces of feedback are overlooked.
The benefits that AI brings to OSINT can hardly be overstated—it enhances processes across the board. But the overarching advantages are threefold:
The amount of data processed during an open-source investigation is usually limited by the number of analysts involved in the task, and the sum total of their brain power and energy levels. However, the scope of AI-driven data processing is restricted only by computing power—in other words, it’s practically limitless.
While specially trained OSINT analysts are still essential for conducting successful investigations with open data, AI tools can dramatically improve the manageability and effectiveness of their work. By highlighting the most relevant information and its structural patterns, analysts can circumvent a lot of the grey noise that tends to accompany the human element in investigations.
Open data has a short shelf-life, and can quickly become out-of-date or vanish entirely. So, quality OSINT work is broadly the result of the analysis that is prompt. In contrast to time-consuming manual processes, AI can extract and organize open data in near real-time. This paves the way for accurate continuous data monitoring, fast pattern identification, instant red-flagging, and even automated reevaluations of entire investigative flows.
At Social Links, we are actively embracing these bleeding-edge AI technologies to develop the most powerful and incisive OSINT tools we possibly can. While it has only been a few months since the headline-grabbing ChatGPT was released, we’ve already harnessed the chatbot to develop a range of new AI-powered text analysis features for our flagship solution, SL Professional. These include:
It can often be the case that, while you are only interested in a particular topic, the text contains multiple themes that are not relevant to what you need. This transformation separates the various topics within a text so you can see at a glance whether it contains relevant content. This can be helpful when you want to aggregate the various topics that might characterize an area of online discussion.
For example, let’s say we have a Facebook group and we want to understand the main topics which get discussed by its members. We can first extract all the individual posts by running [Facebook] Get Posts, then select all of our results and run [ChatGPT] Get Topics. This will not only break down the various topics within these posts but also show how common they are.
This transform analyzes text sentiment in terms of its lexical components, or ‘objects’, breaking down the text into units, which are each assigned a positive, negative, or neutral value. This function can be highly effective in helping you analyze a large number of texts.
For example, let’s say a new law is being passed and you want to know what the public opinion is regarding the legislation, as well as the politicians who are pushing it through. So, we can run a search on Twitter using the name of the new law as a keyword, then assess its reception using this feature.
If you’re dealing with a lot of text, sometimes you just want a very quick assessment that can promptly give you an overall attitude of what has been typed, without needing to read through it all. And that’s precisely what this transform does, returning a result of either positive, negative, or neutral.
This transformation is great in cases when you want to understand the gist of a given text. It analyzes the whole text for you, breaking it down into key points and generating a round-up of its content. For those who work with a lot of text, this function can significantly reduce the time spent on reading.